The rise of AI tools like ChatGPT has sparked a heated debate about how artificial intelligence will transform the job landscape. Will AI eliminate more roles than it creates, or will new tech-fueled prosperity counteract any job losses? Elon Musk and Jensen Huang, two billionaire tech CEOs leading the AI revolution, couldn’t have more different takes.
Their contrasting views, elucidated in recent interviews, provide insight into the optimistic and pessimistic poles of this debate. For Musk, AI represents an existential threat to human employment. But Huang foresees new tech-driven efficiencies spurring business expansion and job growth. Let’s dive deeper into their perspectives.
Musk’s Dystopian Vision: AI Makes Human Workers Obsolete
Musk has repeatedly warned that AI will fundamentally alter society by supplanting human labor. During a talk at the UK AI Safety Summit, he predicted a future where “no job is needed” once AI becomes “smarter than the smartest human.”
This bleak outlook stems from his view that AI systems like ChatGPT will inevitably surpass human capabilities across a broad range of cognitive tasks. As Musk argues, if AI can outperform humans at most jobs, widespread technological unemployment seems inevitable.
Musk believes that even new roles created by AI, like training or monitoring these systems, will eventually be handled better by the AI itself. With human labor no longer essential, people will need to “find meaning in life” unrelated to productive work.
Those who disagree argue this view underestimates humanity’s creativity and adaptability. But Musk remains convinced superhuman AI could make many jobs redundant in the not-so-distant future.
Huang’s Optimism: AI Raises Prosperity and Creates Jobs
In contrast, Huang asserts that AI will be a net job creator rather than job destroyer. Productivity gains from AI, he argues, will make companies more successful and prosperous. And prosperous companies expand by hiring more people to pursue new opportunities.
Huang highlights how today’s industries already employ far more people than those of centuries past, thanks to new technologies enabling the creation of new products, services, and roles. He believes the same dynamic will unfold as AI propels advances across sectors like transportation, retail, healthcare, and more.
This optimistic perspective assumes that economic growth driven by AI will outpace any labor-saving benefits. As long as there are new problems to solve and products to build, human ingenuity and ambition will ensure sufficient demand for human labor.
AI may automate some tasks, Huang concedes, but he believes it won’t stifle business innovation and growth. New tech should create jobs managing, monitoring, and working alongside AI systems.
Key Factors Shaping the AI Job Debate
Digging deeper, several factors help explain these clashing visions from two tech luminaries:
1. Time Horizon
Musk’s warnings focus on a more distant AI future, when systems might exhibit broadly superhuman intelligence. Huang’s near-term optimism assumes today’s narrow AI variants like ChatGPT.
Over a 10-20 year horizon, AI likely generates net job growth as its capabilities improve. But on a 50+ year view, AI could conceptually automate almost any human work.
2. Techno-Optimism
Musk holds a generally techno-skeptical worldview, reflected in his concerns about AI, social media, and other technologies’ societal impact. Huang exhibits greater optimism that technology benefits humanity.
This colors their default assumptions – Musk expects the worst from unrestrained AI, while Huang sees upside.
3. Remote Work Views
Their clashing perspectives on remote work also relate to the AI job debate.
Musk insists that remote work breeds unaccountability and laziness among employees. This bleak view of human productivity aligns with his warnings about AI making human labor redundant.
Huang appears more trusting of remote employees’ work ethic. This matches his optimism about human ingenuity ensuring ongoing demand for human skills even amidst technological change.
4. Leadership & Culture
Finally, their contrasting leadership styles and corporate cultures shape their AI perspectives.
Musk aggressively slashes costs, including workforce cuts, in pursuit of efficiency. His Darwinian tactics reflect his view that only the most high-performing humans can compete with coming AI systems.
Meanwhile, Huang emphasizes avoiding layoffs and sustaining employees’ hopes in Nvidia’s mission. His view that companies should enable human flourishing aligns with his argument for ongoing demand for human skills.
Their diverging assumptions about human nature and the role of business leadership lead to very different conclusions about AI’s impact on the workforce.
What Should Business Leaders Do?
This debate highlights several important implications for business leaders navigating the AI transformation:
- Audit your workforce – Conduct skills audits to identify roles at high risk of automation based on current AI capabilities. But also recognize limitations of today’s AI and where uniquely human skills remain critical.
- Invest in upskilling – Make reskilling and upskilling workers a strategic priority, focused on complementing AI systems and harnessing new opportunities. Lifelong learning mitigates AI’s job displacement effects.
- Question assumptions – Examine your own assumptions about human abilities, creativity, productivity etc. These shape whether you see AI as threat or enabler regarding your workforce.
- Plan for alternative futures – Consider varied scenarios on AI’s evolution and impact over different time horizons. This avoids planning for just one vision of the future.
- Focus on human-AI collaboration – Rather than pure automation, look for opportunities to combine AI and human strengths. This creates new value while keeping workers integral.
The interplay between technological progress and human ingenuity resists simplistic predictions. By accounting for divergent perspectives on AI’s impact, business leaders can make wiser strategic decisions and investments.
The Musk vs. Huang debate highlights why the future of work under AI remains so contested. Their contrasting assumptions lead to starkly different visions on whether AI will eliminate or create roles for humanity. While the reality will likely fall somewhere between their poles, business leaders must grapple with these diverging viewpoints as they plan for AI-driven disruption. Companies able to enhance human potential while harnessing AI’s capabilities will thrive in this new era.
The Most In-Demand AI Job of 2024 Can Pay Over $200,000 and Offers Remote Opportunities
Artificial intelligence (AI) is transforming businesses and job markets around the world. As companies increasingly adopt AI technologies like machine learning, automation, and data analytics, the demand for AI talent has skyrocketed. According to the article, the most in-demand AI job right now is the role of Machine Learning Engineer. This critical position offers high pay, remote work opportunities, and tremendous career growth potential.
In this blog post, we’ll dive deeper into the role of the Machine Learning Engineer, analyze why it’s become so essential, and explore the key skills, qualifications, and responsibilities required. We’ll also look at the impressive salary potential and benefits of remote work that make this the AI job to pursue in 2023 and beyond.
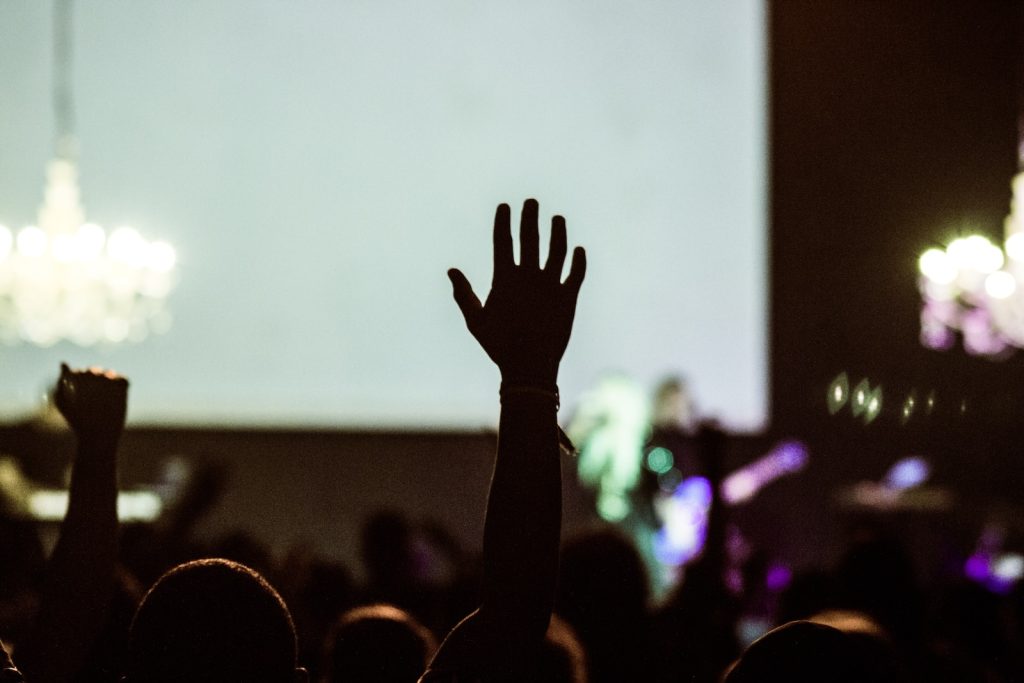
The Rise of the Machine Learning Engineer
Machine learning is a subset of AI that enables computers to improve at tasks and make predictions by learning from data, without being explicitly programmed. Machine learning is powering breakthroughs in areas like computer vision, natural language processing, predictive analytics, and more. As a result, machine learning engineering has become the most sought-after AI skillset.
The machine learning engineer is responsible for building, optimizing, and deploying ML systems to solve business challenges. Their primary duties include:
- Collecting, cleaning, labeling, and preprocessing data for machine learning models
- Selecting and training the most appropriate ML algorithms for a given problem
- Improving model performance through techniques like hyperparameter tuning and ensemble modeling
- Building scalable ML infrastructure to support large datasets and real-time predictions
- Monitoring models in production and retraining as needed based on new data
- Developing APIs and apps to showcase ML model capabilities to business stakeholders
The work of machine learning engineers powers everything from product recommendations to fraud detection, predictive maintenance, personalized marketing, self-driving cars, and much more. Their expertise in statistical modeling and coding is absolutely vital for companies implementing AI.
Why the Demand Has Exploded
Machine learning engineering has emerged as the #1 AI job for several key reasons:
- Shortage of ML Talent: As companies scramble to adopt ML, there simply aren’t enough qualified engineers to meet demand. It’s one of the hardest roles to hire for right now.
- Mission-Critical Nature: ML engineering work enables data-driven automation and insights that provide enormous competitive advantages. It’s a top strategic priority for most companies.
- Rapid Growth of Big Data: ML models thrive on abundant, high-quality data. The explosion of big data from IoT, mobile, social media, and more provides ample fuel.
- Advancements in Algorithms: New techniques like deep learning have dramatically improved ML model capabilities in recent years.
- Cloud Computing Power: The availability of scalable GPUs and compute resources in the cloud has made complex ML modeling more accessible.
- Rising Investment: Funding for AI startups has surpassed over $60 billion globally. All this investment needs ML talent to succeed.
The bottom line is that machine learning engineering is no longer a niche subfield. It has become a mandatory capability for organizations to survive and compete in the digital age. Companies are desperately seeking more ML experts to join their teams.
Sky-High Salaries of $200K or More
All this hunger for machine learning engineers has naturally driven salaries to eye-popping levels. According to recruiting firm Harnham, machine learning engineers in America can expect an average base pay of $146,000. Total compensation at top tech companies like Google and Facebook can exceed $230,000 for those with a few years of experience.
Even at mid-size and smaller firms outside of big tech, six figure salaries above $100,000 are becoming the norm. Machine learning engineers earn a premium for their statistically rigorous computer science backgrounds combined with specialized AI skills. Beyond the healthy base pay, many of these roles also provide equity options that could appreciate greatly.
The earning potential reflects how urgently companies need more ML engineering talent. It’s motivating more technology professionals to skill up and transition into this rewarding AI career path. Given the trajectory of machine learning adoption, these lucrative salary levels should continue rising in the years ahead as demand outpaces supply.
Remote and Hybrid Work Opportunities
Another major benefit of machine learning engineering roles is the abundance of remote and hybrid work opportunities. Employers today are much more flexible about enabling ML engineers to work from home full-time or split time between office and home.
Some key advantages of remote ML engineering work include:
- Less Commuting: Avoid tiresome commutes and regain productive hours each day.
- Family Time: Remotely gives much more flexibility for family responsibilities like childcare.
- Flexible Hours: Have a more flexible schedule that fits your personal chronotype and habits.
- Reduced Costs: Save money on gas, car costs, buying lunch, wardrobe, and more.
- Comfort: Stay comfortable in your home environment without distractions from chatty coworkers.
- Safety: Avoids health risks of being onsite during illnesses like COVID.
- Productivity: Many engineers report higher productivity and creativity when coding from home.
- Geographic Mobility: Puts you in reach of employers located anywhere, not just locally.
The technical nature of machine learning engineering allows much of the work to be conducted remotely. Leveraging tools like GitHub, Jupyter Notebooks, and cloud platforms enable effective collaboration without being co-located. The hybrid model is also ideal for striking a balance between focused remote work and in-person team interactions.
Of course, being fully remote also has some tradeoffs to consider around communication, company culture, and socialization. But for many, the benefits now clearly outweigh the downsides. The AI field is ahead of the curve in embracing remote work, so take advantage of the opportunities.
Requirements and Qualifications
Transitioning into a machine learning engineer role can be challenging. To land one of these coveted jobs, you’ll need the right mix of work experience, technical skills, and education. Here are the key requirements to get started:
- Education: A bachelor’s degree in computer science, software engineering, math, physics, or statistics is essential. A master’s degree or Ph.D is preferred by some employers.
- Programming: Proficiency with languages like Python and R is mandatory. Experience with libraries like NumPy, Pandas, Scikit-Learn, PyTorch, and TensorFlow is a huge plus.
- Math: A strong foundation in calculus, algorithms, probability, multivariate statistics, and linear algebra is expected.
- Cloud Platforms: Knowing how to operationalize models with AWS, GCP, Azure, etc. is valued.
- Software Engineering: Production ML systems require solid software engineering abilities and tools like Git, CI/CD, Docker, etc.
- Problem Solving: Strong critical thinking and analytical problem-solving is vital for ML engineering.
- Communication: You’ll need to synthesize complex topics and collaborate cross-functionally.
- Production ML: Prior experience deploying real-world ML systems is highly desired, though entry-level positions exist.
Meeting all these prerequisites can take years of diligent education and work experience. Competition is stiff for machine learning roles, so set yourself apart by mastering the material and building an impressive portfolio of ML projects.
Moving into management and leadership roles will require additional skills like people management, product sense, and executive communication. But the opportunities for career growth in this field are immense.
Conclusion
Elon Musk and Jensen Huang provide two influential, yet sharply conflicting outlooks on AI’s implications for the workforce of the future. Their techno-pessimism versus techno-optimism encapsulates the swirling uncertainty and debate regarding human labor’s role amidst accelerating AI progress.
By considering their perspectives, business leaders gain crucial insights to navigate through the AI-driven turbulence ahead. With wise investments in upskilling workforces and creatively combining human and artificial intelligence, companies can build a future where both technology and humanity flourish in tandem.
Machine learning engineering clearly represents an enormously promising career path today. The soaring demand, six-figure pay potential, and flexibility of remote work make it the most coveted AI job role out there. Breaking into the field requires dedication to mastering advanced skills, but the rewards will be well worth it.